Machine Learning in Endpoint Protection- A New Era of Cybersecurity
- Shiksha ROY
- 3 days ago
- 4 min read
SHIKSHA ROY | DATE: JANUARY 14, 2025

As cyber threats grow increasingly sophisticated, traditional endpoint protection methods are often inadequate in identifying and mitigating advanced attacks. Machine learning (ML) is transforming the landscape of endpoint security by offering intelligent, adaptive, and proactive measures. This article delves into how machine learning is reshaping endpoint protection and why it represents a new era in cybersecurity.
Understanding Endpoint Protection
What is Endpoint Protection?
Endpoint protection refers to the security measures deployed to protect devices such as laptops, desktops, servers, and mobile devices that connect to a network. Traditional methods rely heavily on static signature-based detection, which identifies known threats but struggles against new and evolving attacks.
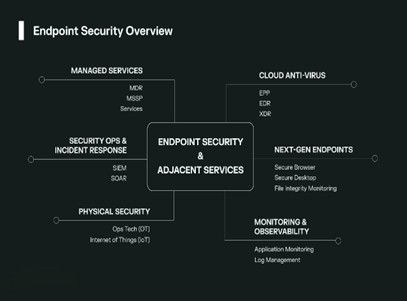
Traditional Endpoint Protection Methods
Historically, endpoint protection relied on signature-based detection, where known malware signatures were used to identify threats. While effective against known threats, this method struggled with zero-day attacks and sophisticated malware that could evade detection.
The Role of Machine Learning in Cybersecurity
Machine learning is a subset of artificial intelligence that enables systems to learn and improve from experience without explicit programming. In endpoint protection, machine learning examines large datasets to identify patterns, anomalies, and potential threats in real time.
Key Features of Machine Learning in Endpoint Protection
Behavioral Analysis
Machine learning models monitor user and device behavior to identify deviations from normal patterns. For example, if a device suddenly starts transmitting large amounts of data to an unknown server, ML algorithms can flag it as suspicious.
Threat Prediction
By examining past data, machine learning can forecast possible vulnerabilities and threats, allowing for proactive security actions.
Anomaly Detection
ML algorithms excel at detecting anomalies, such as unusual login locations or irregular access patterns, which might indicate a security breach.
Advantages of Machine Learning in Endpoint Protection
Real-Time Threat Detection
Unlike traditional methods, which may require frequent updates, ML continuously learns and adapts to new threats in real time. This ensures that organizations remain protected against zero-day attacks.

Reduction in False Positives
Static security systems often generate false positives, overwhelming security teams. Machine learning minimizes these by accurately differentiating between legitimate activities and potential threats.
Scalability
Machine learning solutions can analyze and process vast amounts of data, making them suitable for large organizations with extensive networks.
Automation and Efficiency
ML-powered endpoint protection automates threat detection and response, reducing the reliance on manual interventions and enabling faster resolution.
Applications of Machine Learning in Endpoint Security
Malware Detection
Machine learning can identify malware based on its behavior, code structure, and execution patterns, even if it has never been seen before.

Ransomware Prevention
By analyzing file behaviors and encryption patterns, ML algorithms can detect and block ransomware attacks before they encrypt critical data.
Phishing Detection
ML models can analyze email content, sender behavior, and attachment characteristics to identify and block phishing attempts.
Insider Threat Detection
Machine learning helps monitor and analyze employee activities to detect potential insider threats, such as unauthorized access to sensitive data.
Challenges of Machine Learning in Endpoint Protection
Data Dependency
Machine learning models need extensive, high-quality data to perform optimally. If the data is poor or biased, it can result in inaccurate outcomes.
Evasion Techniques
Cybercriminals continuously develop techniques to evade ML-based detection, such as adversarial attacks that manipulate input data to deceive algorithms.
Resource Intensity
Training and deploying ML models can be resource-intensive, requiring significant computational power and expertise.
The Future of Machine Learning in Endpoint Protection
As cyber threats evolve, machine learning will play an even more critical role in endpoint protection. Future advancements may include:
Deep Learning Integration
Leveraging neural networks to analyze complex data patterns with greater accuracy.
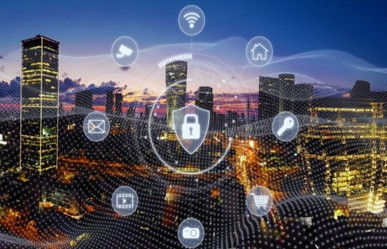
Federated Learning
Allowing decentralized devices to collaboratively train models without sharing sensitive data, enhancing privacy.
Self-Healing Systems
Using ML to automatically repair vulnerabilities and restore compromised systems without manual intervention.
Conclusion
Machine learning is revolutionizing endpoint protection by providing dynamic, intelligent, and adaptive defenses against modern cyber threats. While challenges remain, its ability to detect and respond to attacks in real time, minimize false positives, and scale across large networks makes it indispensable for the future of cybersecurity. By embracing machine learning, organizations can strengthen their security posture and usher in a new era of robust endpoint protection.
Citations
CrowdStrike. (2024, April 15). CrowdStrike’s approach to artificial intelligence and machine learning. crowdstrike.com. https://www.crowdstrike.com/falcon-platform/artificial-intelligence-and-machine-learning/
Machine Learning meets endpoint Security: the future of predictive threat Detection. (n.d.). https://hcl-software.com/blog/bigfix/machine-learning-meets-endpoint-security-the-future-of-predictive-threat-detection
What is Endpoint Security? Definition, key components, and best practices. (2025, January 7). Spiceworks Inc. https://www.spiceworks.com/it-security/network-security/articles/what-is-endpoint-security/
Megasis Network. (2024, November 30). AI in Endpoint Security: Protecting Devices from Advanced Threats. Medium. https://megasisnetwork.medium.com/ai-in-endpoint-security-protecting-devices-from-advanced-threats-d1a6a6738ba3
Kaya, Y., Chen, Y., Saha, S., Pierazzi, F., Cavallaro, L., Wagner, D., & Dumitras, T. (2024, May 9). Demystifying Behavior-Based malware detection at endpoints. arXiv.org. https://arxiv.org/abs/2405.06124
What is machine learning in security? (2023, November 9). Cisco. https://www.cisco.com/c/en/us/products/security/machine-learning-security.html
Columbus, L. (2019, September 25). 10 Ways AI and machine learning are improving Endpoint Security. Forbes. https://www.forbes.com/sites/louiscolumbus/2019/09/25/10-ways-ai-and-machine-learning-are-improving-endpoint-security/
Image Citations
Columbus, L. (2019, September 25). 10 Ways AI and machine learning are improving Endpoint Security. Forbes. https://www.forbes.com/sites/louiscolumbus/2019/09/25/10-ways-ai-and-machine-learning-are-improving-endpoint-security/
InfoSec (www.infosec.gov.hk). (2025, January 14). InfoSEC: Ransomware. InfoSec. https://www.infosec.gov.hk/en/knowledge-centre/ransomware
What is Endpoint Security? Definition, key components, and best practices. (2025, January 7). Spiceworks Inc. https://www.spiceworks.com/it-security/network-security/articles/what-is-endpoint-security/
Harrison, K., & Odum, F. (2022, November 30). The era of endpoints. https://research.contrary.com/deep-dive/era-of-endpoints
Comments